Increasing Use Of Artificial Intelligence By SMEs
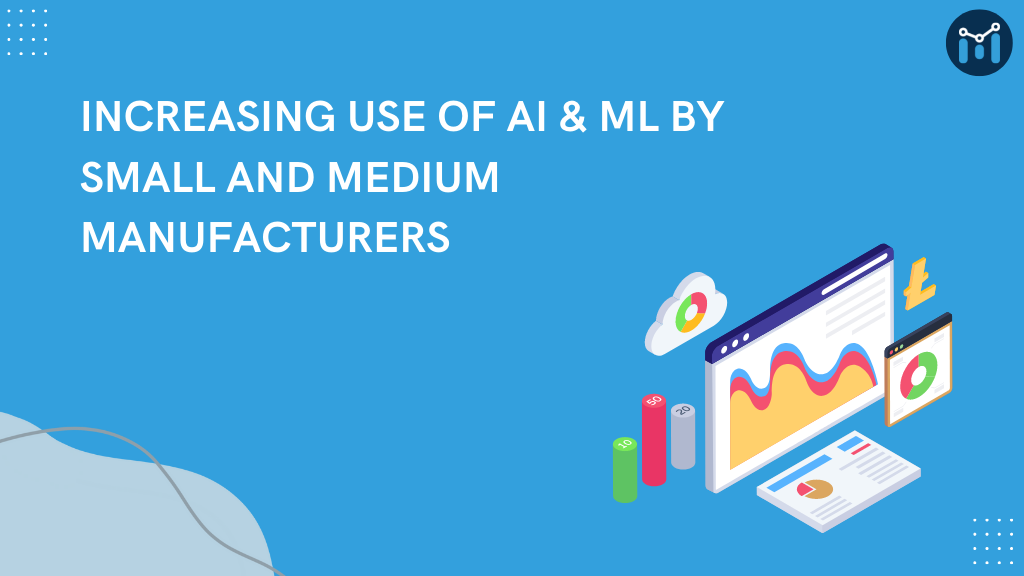
The manufacturing industry is no longer dependent on traditional methodologies in production. The research indicates, this is the second largest industry looking towards the advanced technologies such as Artificial Intelligence and Machine Learning in Manufacturing to fix their production issues like lower production, poor quality, and higher manufacturing cost.
As per another survey by Global Market Insights, global AI in manufacturing is anticipated to grow up to $16 billion by 2025. It makes sense for the process manufacturing, which is a highly competitive sector with swiftly-changing markets and complex systems. The combination of AI and ML tools in manufacturing help companies take a deeper look into their logistics, inventory, assets, and supply chain management. There are several AI use-cases in manufacturing that proves the fact. Let us learn more about why we are witnessing the increasing use of AI/ML by small and medium manufacturers.
1- Predictive Maintenance
Unplanned downtime costs heavy to plants and factories. A survey found unplanned downtime to cost nearly $50 billion. Out of which, 42% of the cases were due to asset failure. Factories running on older methodologies perform maintenance according to a predetermined schedule or using SCADA (Supervisory Control and Data Acquisition) systems set up with human-coded thresholds to prevent unplanned downtime. However, these techniques add cost and time to the company. For example, the company has to go for maintenance on a scheduled time whether the machines need repairing or not. Similarly, the SCADA system demands frequent updates to add changes to the tags, units of measure, scripts, alarm thresholds, etc. And also go for frequent reboots for security purposes. No wonder why such manual approaches are seeing a long-term decline over the time:
On contrary, predictive maintenance uses pre-designed algorithms to predict the next failure of a component/machine/system. Hence, the personnel get the alerts to perform focused maintenance procedures only when the machines actually need it and not too early.
This is just a very basic reason for small to medium enterprises adopting AI/ML in their factories on a larger scale. There are numerous other advantages of AI/ML over the traditional manual and semi-manual approaches. For example:
a) Unlike traditional approaches, AI/ML-based predictive maintenance can consider the more complex dynamic behavioral patterns of the machinery system as well as the contextual data in relation to the manufacturing procedure. For instance, if a sensor on a production machine observes and reports a sudden rise in temperature, there can be several reasons for the case. Predictive analysis will analyze the situation and understand the fact that the machine is undergoing sterilization. On the other hand, a static rule-based system would not consider any other possibility and proceed to trigger a false-positive alert.
b) By detecting a possible failure in advance with a machine learning algorithm, production systems can continue to function without unnecessary interruptions, saving cost and time in manufacturing.
c) The personnel are very well informed in advance about the segment demanding repair. The maintenance is very focused as technicians know about the components that need inspection, repair and replacement. Also, they are aware of the tools to use and the methods to follow, hence significantly reducing the maintenance costs while eliminating the need for planned downtime in many cases.
d) Predictive maintenance ensures a longer Remaining Useful Life (RUL) of machinery and equipment. This is because it can prevent secondary damage and hence would need only smaller labor forces to perform maintenance procedures.
2- Predictive Quality
Maintaining the quality of the product is a challenging task for any manufacturer when technical faults in the machinery function are a usual threat. But with AI, this issue can be handled very well. Predictive Quality is one of the advanced benefits of Industrial Artificial Intelligence. Manufacturing companies are largely using AI for quality control in their plants.
It reveals the hidden causes of many repeating process-based production losses that manufacturers come across on a regular basis. The losses can be of quality, yield, throughput, waste, energy efficiency, emissions, or anything caused by process inefficiencies. Predictive Quality can efficiently find out the actual causes behind the process-driven production losses using Machine Learning – one of the most common subsets of AI. Machine Learning algorithms perform continuous, multivariate analysis, and therefore can deeply understand each individual production process. Once ML identifies the cause of the losses, an automated recommendation program and alerts system can be used to inform production teams and process engineers about the imminent loss as well as the information needed to prevent the losses in advance. Consequently, using industrial AI in factories becomes a necessity for manufacturers.
3- Predictive Output
Another very popular use case of AI in manufacturing is predictive output. A lot of data is collected throughout the production process, which can be processed, analyzed, map the function, and predict the output for each round of the production process. But not all plants have the system to utilize the data. As per the survey, manufacturing companies discard 98% of all the data they can collect due to the lack of operational analytics capabilities to integrate the collected data into their operations. However, supervised machine learning is emerging as one of the widely accepted techniques to get a predefined output target. The technique requires data input, data training, defining and choosing the right algorithms, data visualizations, and more. The idea is to construct an accurate mapping function to predict outputs when new input data is entered into the system. The algorithm is created using a training dataset and after working through iterations to continuously improve its performance to reach the defined output.
4- Market Adaptation
Artificial intelligence-based applications can largely help in analyzing the market fluctuations or changes. Hence, the suggestions by AI can be further used to improve business strategic vision. The process of AI analysis for market change considers several factors, such as political scenarios, weather conditions, consumer behavior, and the economic status of a particular region. Each and every factor is evaluated deeply so that managers can improve supply chains and make their strategies interact with changes in the market beforehand. With the suggestions and predictions offered by the AI-based applications a floor manager can measure staff, inventory, and the supply of materials.
5- Limiting Expensive CT Scanning
Every round of additive production bears some uncertainties. This is why using NDT (non-destructive testing) becomes necessary. However, NDT is expensive, especially when there are CT scanners for analysing the structural integrity of manufactured parts. The cost of testing can be reduced by linking sensors in the machines to AI applications. The data collected from sensors are real-time information that can be used to build a machine-learning model. For an instance, sensor data can flag parts that the analytic model suggests to be defective and do not require the part to be CT-scanned. Therefore, only those specific parts would be scanned instead of scanning the entire part.
6-Generative Design
Generative design is an essential use case of AI in manufacturing for saving time for design engineers and labour to create repeated designs.
It works in a simple way. A design engineer enters a set of requirements of a project into design software, which creates multiple iterations of the design process.
The most popular advantages of the generative design are as follows:
a) Generative design is perceived as an adaptable optimization technique. This is because an effective generative design algorithm has the ability to understand how a manufacturing process impacts the individual features and geometry of a material, leading to the change in material properties.
Based on its, analysis, it creates a process to repeat the specified design.
b) Another advantage of generative design is that manufacturers can use it for manufacturing products at facilities/plants in different locations.
The generative design application produces optimal design and specifications. Thereafter, it distributes the design to multiple facilities with compatible tooling. As a result, smaller, geographically dispersed plants can produce a larger range of parts.
It is a highly sought-after feature in the aerospace and automotive industry which require producing parts for vehicles and the next day making parts for other essential products to save distribution and shipping costs.
7- Industrial Robotics
In 2020, spending on robotics and drones was nearly is about USD128.7 billion in total and is expected to grow further in coming years. Manufacturing industries are widely using robotics in their plants and facilities to improve production line function and reduce wastage of resources. With the number of advantages robotics offer to this industry, its popularity is evident among small and medium factories. Industrial robots efficiently automate repetitive tasks and prevent or minimize human error nearly to a negligible rate. Moreover, like an AI-based industrial robot, these tools can monitor its own accuracy and performance, and train itself to improve its function. Some of the advanced manufacturing robots are equipped with machine vision. This feature enables the robot to achieve the required mobility in complex and random environments. Machine vision precisely works safely alongside human workers to accomplish a task that cannot be fully automated and are unsafe to work directly with. Hence human workers can shift their focus to more productive areas of the manufacturing operations.
8- Demand Forecasting
Demand forecasting is primarily a branch of predictive analysis. In this process, the customer demand forecast is estimated by analyzing historical data. For small and medium factories that always strive to match the future product demand, AI turns out to be a great solution for them. It is emerging as a successful solution, which is why demand forecasting is the most widely used machine learning application in supply chain planning. As per the study, 45% of companies are already using the technology and 43% of them are planning to use AI-powered demand forecasting within two years. Although traditional demand forecasting methods are also there like ARIMA, exponential smoothing, etc., they don’t provide accuracy.On contrary, AI-powered demand forecasting tools offer full accuracy while managing inventory levels better, preventing the possibility of cash-in-stock and out-of-stock scenarios.
Please read our two blogs wo gain more knowledge on AI and how MES can benefits SMEs.
Ground-Breaking Benefits Of AI And ML In Manufacturing Industry
Manufacturing Execution System: Buyers’ Guide for Small and Medium Enterprises
Summary
ML (Machine Learning), is the most common subset of Artificial intelligence that allows robots and personnel to collaborate to accomplish tasks quickly and with more accuracy. Manufacturers always struggled to reduce losses in the production process. But now with Industrial AI/ML-based tools, achieving this mission has become easy like never before. AI makes machines smarter so they can take on more and more repetitive tasks. The idea is to improve speed, precision, and quality control in manufacturing, as well as allow human counterparts to spend more time solving other problems. While AL was always popular among large enterprises, the continuously surging market competition is also pushing small and medium enterprises to adopt the technology. The popularity of AI has gained momentum in recent times also due to the availability of cost-effective AI solutions.